Why picking the right AI-credit decisioning partner matters
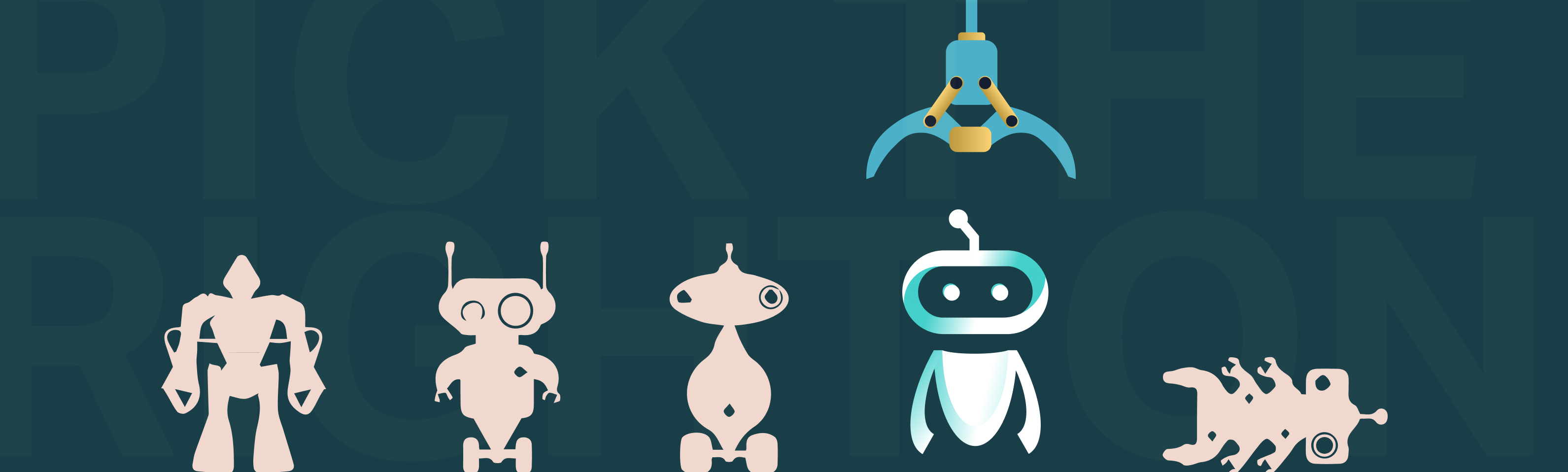
AI and enhanced lending practices go hand-in-hand — the technology you choose to implement will make the difference for a better underwriting experience
As financial institutions continue to evaluate AI underwriting solutions to incorporate into their lending strategies, recognizing the distinction between vendors and true partners in innovation is more important than ever. While a vendor offers a technological solution, partners additionally provide ongoing support in strategizing, ensure compliance is held to a higher standard, and align with organizational values.
So, you know what you get when you pick the right AI underwriting partner — but why does it matter that you find the right one? Ultimately, it’s because you want to run your business better, faster, and more fairly.
ISO: data scientists and AI experts!
In today’s world, filled with every kind of AI start-up imaginable, it can be pretty difficult to find (and hire) AI experts and data scientists. In fact, in a report on the state of AI in financial services, NVIDIA found that one of the top challenges, at 36 percent, for lenders was recruiting and retaining data scientists. And that’s the institutions that can afford to hire data science teams — a data scientist’s salary can rival that of doctors and other medical practitioners. Small or even large lenders may not have the capital to invest in subject matter experts, and they certainly don’t have the time to create the necessary technology when, if you don’t mind a simile, the US has already landed on the moon.
So, it’s hard to hire AI experts, or you’re spending a lot of money and time on building AI banking tools? In that position, I’d want a third option. I’d like to know who’d won “The AI Race” and be curious to see how they did it.
Zest AI landed on the moon (built an AI-automated lending tool) back in 2009, has retained a team of data scientists and AI experts to help continue to improve our technology, and wants to help you understand exactly how AI can help your business make smarter, more inclusive, and efficient lending decisions.
Why does an AI underwriting partner make your lending business better? You avoid internal talent shortages, have access to industry-first features, and the expertise and resources needed to get a model through compliance and regulatory review, integrate it with your existing LOS, and keep up with today’s breakneck pace of innovation.
Be paired up with a partner who can help you successfully navigate changing times before they happen
As established in the prior section — Zest AI’s humble beginnings trace back to 2009, so we were a little late to the scene to help navigate the Great Recession. But we took a little trip back to the mid-2000s to see if our technology could stand the test of time. You might find yourself saying, “Wait a minute, Doc, are you telling me you built a time machine out of a technology company?” in response to that statement, and we’re really joking… about the time machine part.
Zest AI’s loan underwriting fared substantially better than the national scoring method netted out in 2008 because our technology uses more data and better math to predict risk. And that lesson in navigating a financial crisis positively influenced our machine learning’s ability to predict risk in uncertain economic climates. As did our experiences working with lenders through the COVID-19 pandemic. But we’ve always been concerned with keeping up with the economic outlook and ensuring that our technology is prepared to keep your lending business on track. We like to see our clients thrive, and when we can do that through an economic downturn, well, that’s just a cherry on top of the sundae that is our partnership.
Working with a trusted AI partner who’s made it their mission to have your back means that your organization not only has an internal team that’s ready to take on shifts, changes, and turns but an external set of hands that has their eye on the economy, learnings from across a vast client base, and the ability to learn from the past to build a faster response to any future we face.
The right partner can help automate your compliance practices
Fair lending practices are no joke — and we here at Zest AI take compliance very seriously because we believe that a better, richer, and fuller life is available to all if we can build a system that supports equitable access to credit.
The financial services industry has laid the blueprint for legislating AI for other highly regulated industries. That work has been paid through years of navigating and correcting systemic issues within the financial services industry. Through machine learning advancements, adversarial debiasing better predicts the risk of borrowers, enabling more loan approvals — 40 percent to be exact — for protected class persons seeking out credit. The right AI partner promotes an understanding of how to use AI in underwriting correctly, builds products that promote equity, and raises the standards for what it looks like to lend more fairly.
Choosing the right partner isn’t just a means of making decisions more quickly and building in automation for your workflows — it can also strengthen your compliance efforts and enable better, fairer credit decisions across your lending products. But that partner needs to understand the technology they’re building and abide by the laws that govern fair lending. Without these two elements, you’re potentially working with a technology perpetuating unfair lending practices.
You can pick your friends. You can pick your technology partner. But you can’t pick your friend’s technology partner. This is true — and we believe you should be making a fully informed decision when investing in new technology. So the “why” of it all matters. Technology, for technology’s sake, isn’t going to help you in the long run, and starting up now with the right partner can save you time and money down the road.