Improving this algorithm can make lending a lot less racist
There’s a bug in the technology of the US credit economy, one that is possibly holding back people of color from building real wealth
The bug in question is an algorithm called BISG, or Bayesian Improved Surname Geocoding, which many banks and credit unions use in their fair lending analysis to estimate a borrower’s race based on their last name and location.
By law, lenders have to analyze their portfolios regularly to ensure they’re not discriminating based on race, gender, and a range of other protected classes. But only mortgage lenders are allowed to gather borrower race data. Everyone else has to rely on techniques like BISG that estimate race based on non-race associated data.
The problem with BISG is that it’s often wrong, which creates an untold impact on millions of Americans
Using a crooked yardstick to assess the racial disparity of loan approvals can provide lenders with false confidence that their credit models are much fairer than they are. Not understanding where real disparity occurs makes it impossible for the lender to identify problematic lending policies and for the regulator to assess the harm. As a person of color and a data scientist, I felt doubly compelled to do something about it and build a better algorithm.
I’m a perfect example of how BISG gets it wrong. I live in Glendale, California — one of the whitest cities in America. Only 0.3 percent of Glendale residents are Black.
With a name like Kasey Matthews, and a home in Glendale, guess who thinks I’m White? BISG.
When I ran my name and zip code through BISG, it guessed that I had a 90 percent chance of being White. That means that all my good credit behavior, or that of anyone like me living anywhere else like Glendale, would get mischaracterized as that of a white person by a lot of fair lending analysis. This might not seem so problematic on its face, but because I’m counted as White, if my loan application gets declined, that decline is counted as a White decline not a Black decline. A 2014 Charles River Associates auto lending study, found that BISG correctly identified African-American borrowers a mere 24 percent of the time at an 80 percent confidence threshold. Hispanic and Asian borrowers were correctly identified 77 percent and 60 percent of the time, respectively. At a 50 percent confidence threshold, BISG was no better than a coin flip for Black borrowers.
The CFPB, using a different set of loans, found that BISG correctly identified only 39 percent of African Americans. “These differences highlight just how wide-ranging the error rates can be based on the populations,” said the CRA report’s authors. The report goes on to argue that because of the inaccuracy of the BISG analysis method, harm identified in fair lending analysis may be overstated. It is no surprise that one US House lawmaker called BISG “junk science.”
To be fair, BISG wasn’t intended for use in fair lending analysis
It was developed by the Rand Corporation in 2000 to help determine whether minorities were receiving healthcare at the same rate as Whites. In small geographic segments, especially in racially or ethnically homogenous areas, Rand believed BISG was right nine out of 10 times in identifying people as African American. Eventually, the CFPB adopted it for judging lender outcomes and has levied millions of dollars of fines for racial bias, many of which were based on BISG.
Statisticians have tried to improve BISG. A variant called BIFSG added first names to the mix. Another method predicts ethnicity based on a name’s character sequence. Neither moves the needle much on accuracy. Consumers deserve better, as do the lenders and regulators who make the decisions that affect the lives of millions of borrowers.
Earlier this year, the Zest AI data science team built a new neural network called Race Predictor that, in a test on Florida voter data, outperforms BISG by 60 percent, correctly identifying African Americans 74 percent of the time compared with 47 percent for BISG. Race Predictor correctly identified Hispanics 87 percent of the time, compared to 77 percent for BISG. While there is plenty of work to be done to make it better, and we welcome help from partners, Race Predictor is showing promising results.
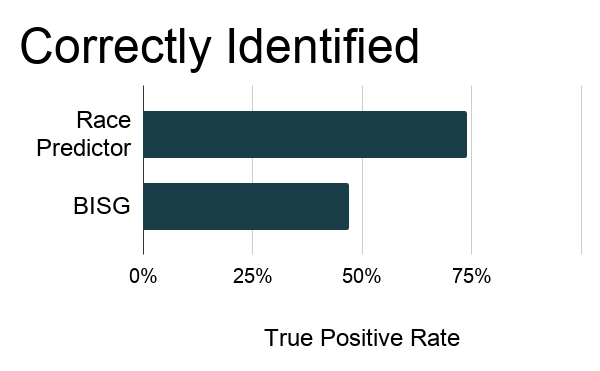
Race Predictor is also better than BISG at delivering true positives with high confidence that hold across more diverse groups (see charts below). By contrast, BISG is almost never certain about a person’s race unless they’re White.
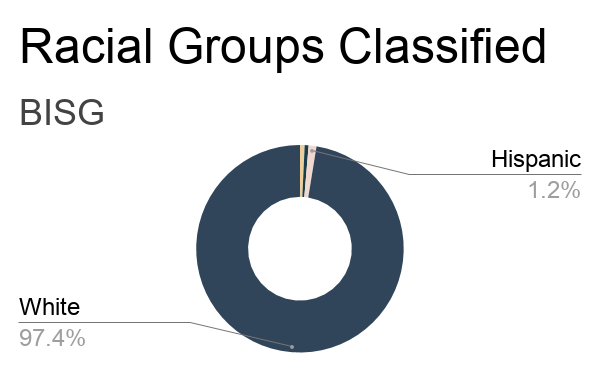
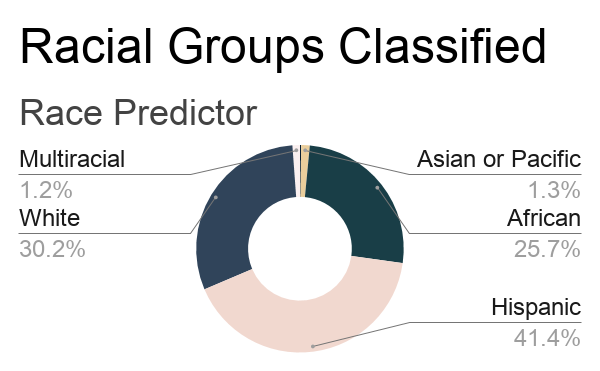
Zest Race Predictor is a natural extension of BISG
It uses the name and address information and adds other race-correlated data such as the US Department of Agriculture’s atlas of community food access and Environmental Protection Agency stats on neighborhood walkability.
To make the best use of the additional data, we’ve replaced the simple Bayesian statistical method used to create BISG with modern machine learning methods that Zest and sophisticated US lenders employ for credit underwriting. These techniques are proven in credit underwriting where they help our customers become more profitable. With Zest Race Predictor, we are applying these advanced methods to provide benefits to those that are underserved.
Zest Race Predictor was trained and validated on roughly a million people from several Florida counties via the Florida voter database, one of the largest publicly available sources of demographic data that includes name, address, and ethnicity. A model trained only on this subset may not generalize to a national population, but it’s a solid place to start. We’d love to use national race data, but the US Census doesn’t make that data publicly available.
We plan to update Zest Race Predictor later this year to improve its accuracy with more data sources, geographies, and new machine learning techniques.