Sowing the seeds of successful loan growth
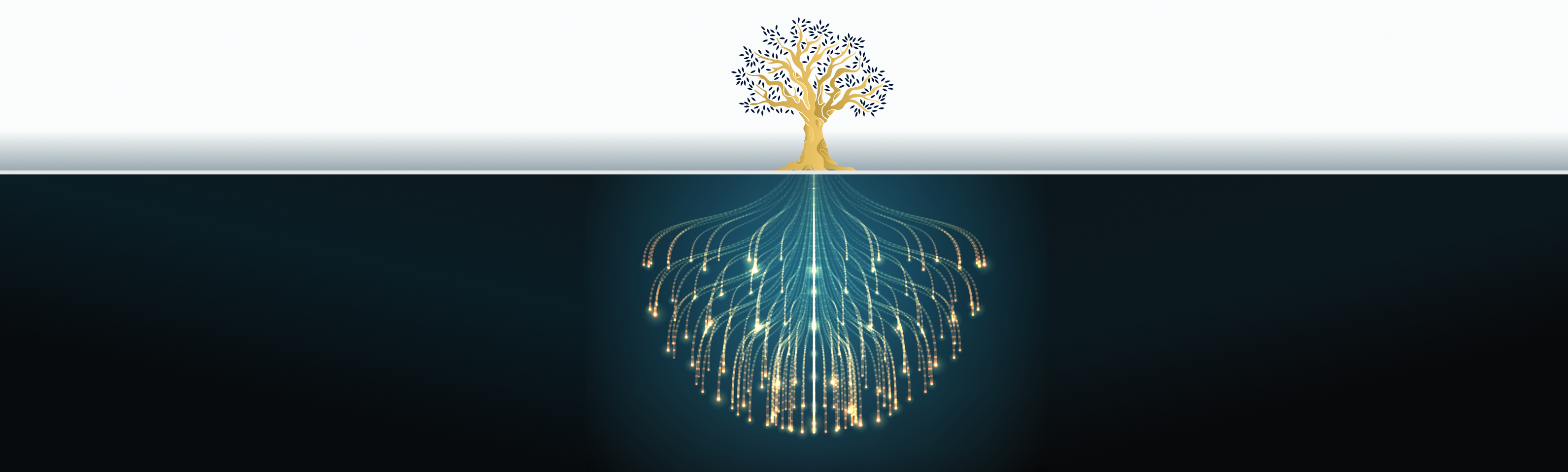
Machine learning helps underwriters grow, safely
So, maybe it’s about time to get our heads out of the sand and talk about what we’ve seen happening in the banking industry. We’ve been on high alert since March 2023, when we watched Silvergate Bank, Silicon Valley Bank, and Signature Bank collapse, and then the takeover of First Republic Bank by JPMorgan Chase a few months later. The CFPB is — rightly — working to ensure that fair lending practices are being applied to AI in the industry, and President Biden signed an Executive Order calling for the prioritization of privacy and civil liberties when implementing AI into all industries. The financial services industry is vulnerable, and industry folks have grown wary of using AI technology, even though the banking industry paved the road for regulating the use of AI for years now.
Should lenders be thoughtful about what kind of AI-driven lending technologies they choose? Obviously. But to be wary of all AI technology means that lenders will fall behind those who take advantage of innovation. When it comes to AI-automated underwriting, we’re here to help.
In a world where lenders are feeling vulnerable due to shifting economic conditions and are looking for transparent, efficient recession-proof lending technologies, AI credit decisioning rises in the ranks of affordable value-adds to give your institution a safe path for growth.
How to grow loans with machine learning as your fertilizer
Let’s get some synonyms out of the way here, because the naming system for AI and credit decisions is almost as complex as the math itself. When we’re talking about AI-automated underwriting, AI credit decisioning, or AI underwriting — it’s the same thing. And since machine learning is a subset (though broad) of AI, it’s always good to note that machine learning credit models also fall under that spectrum of synonyms.
Machine learning helps plenty of lenders solve an ever-elusive problem — how do you enable safe loan growth? With that question, a financial institution has two main paths to follow — increasing approvals while keeping risk constant, or reducing risk while keeping approvals constant.
You want to increase approvals and hold risk constant?
AI-automated underwriting has the capability to increase your approvals by 20 percent, or more, without changing your institution's risk tolerance.
It does this by finding new borrowers who are often overlooked by current credit scoring techniques and adding them into your pool of available borrowers. A low credit score — especially from an institution that ranks credit risk on a five-tier scale — doesn’t always mean someone's high risk for loan default.
These folks are out there, especially among populations with a thin credit file or a once-blemished credit history. But they deserve a shot at credit and machine learning does a great job at finding those skipped-over individuals by using better data and analytics to more accurately predict risk and include folks who fit your criteria for receiving a loan.
You want to decrease risk, while keeping your approvals constant?
Machine learning offers better credit risk assessment to institutions looking to reduce risk instead of growing their lending pool, and AI credit decisioning can often reduce risk by up to 15 percent. For most borrowers, traditional credit scores are a pretty good indicator of their ability to pay back a loan or credit card debt. But in every credit risk tier, there are borrowers who may be riskier or safer than their credit score suggests. Think of how traditional scoring would assess these two individuals: a 25-year old marketing manager who recently gave up his steady paycheck to pursue an acting career, or a doctor who recently emigrated to the US to practice medicine, but lacks an established credit record. No judgment on life choices here, but the likelihood of the 25-year old getting a loan over our thin file doctor is pretty high.
Enter machine learning. AI excels at evaluating risk at the margins — which is where new customers reside and losses lurk. Machine learning can actually predict credit risk of 90 percent of those folks that traditional credit scores deem “unscoreable.” Meaning our doctor can be risk assessed. But our new actor also has a better risk assessment, too, through the use of trended data evaluation. Landing a gig or not, AI loan decisions are better predicted across the spectrum.