So, you’ve made a credit decision… Can you explain it?
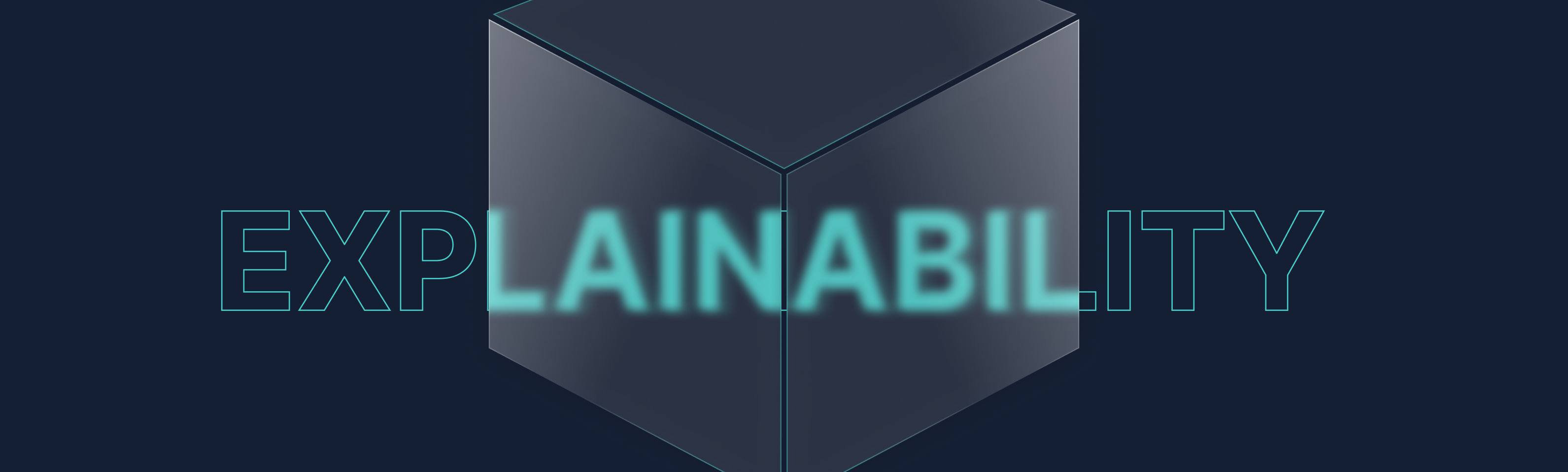
Why explaining model decisions is crucial to fair lending
Lenders of all sizes have embraced machine learning for credit underwriting because of its proven track record of being the most accurate and effective way to enhance fairness for borrowers.
In light of the CFPB’s continued guidance for the use of AI in lending decisions and President Biden’s October executive order that includes key directives on equity, consumer protection, and AI governance, we thought it was the perfect time to go over best practices and nuances that come with model variations and to lay out some key elements behind how model explainability works.
As the CFPB’s Director, Rohit Chopra, rightly put it, “Creditors must be able to specifically explain their reasons for denial. There is no special exemption for artificial intelligence." Providing the basis for fair lending, explainability is crucial when a credit decision is a “no” instead of a “yes.” Adequately explaining a credit denial starts with the data.
Explainability goes data-point-deep
When it comes to rooting out bias and promoting fairness, we know that machine learning models for underwriting can pass the test. We can first look at how models are built. Adversarial debiasing is one method applied when training models to help them continue to predict risk accurately and fairly.
But then we go deeper because it is equally essential to ensure that underwriting models don’t rely on unlawful attributes, or data that proxies for a protected class status, to make their decisions. Being able to confirm that every data point used by your model, as well as the inferences they make when combined with other variables, is fair and unbiased goes a long way in ensuring credit decisions are fair and explainable.
This is when we can look at the models’ outcomes. When you can explain each credit decision down to its individual data points, the work you’ve done at a granular level allows you to say “yes “ to more protected-class borrowers without changing your risk tolerance. Because the data used is more accurate, less biased, and better at predicting risk, AI-automated underwriting can open doors for borrowers while protecting lenders.
Using the right tools gives the best explanation
Consider this analogy: your business is selling two products, one is hand-painted vases, and the other is ceramic plates. One is nice to have in your home for a little extra flair, and the other is essential for eating a meal. Now, the vases must be painted similarly enough for the human eye to not notice much difference. The plates, however, must be physically identical to one another to stack and be functional for your customers.
You have a number of tools at your disposal to assess whether each of these products is fit to be sold. A magnifying glass is a tool precise enough to see an obvious crack or smudge in a vase’s design, but not precise enough to assess the quality of the plates. An electron microscope happens to better suit this second task, a powerful and highly specified way to determine the physical attributes of a plate that are unable to be seen with the naked eye. Using the right tool to assess your product enables both quality and efficiency.
To explain how the model makes decisions, you have to know what the right tool is for the job. AI-automated underwriting models are pretty complex since they’re built based on data sets that include numerous variables. So, given the general complexity of a model — and the fact that different variables can give us the same information about a borrower — there's a nuanced approach when it comes to explaining underwriting model decisions.
We can now apply these principles to credit underwriting: The tools at your disposal must be used appropriately — leave the detail of the electron microscope for the high-stakes applications like underwriting, but remember a magnifying glass can still do the trick for low-stake applications. While these tools aren’t interchangeable, they each serve their purpose for the tasks at hand.
Though a magnifying glass may have been the tool of choice in the past, the level of scrutiny a microscope applies, sets the standard for AI-automated underwriting today. Models can be trained to use the correct level of scrutiny to clearly and correctly weigh a variable in telling the story of credit risk accurately and efficiently. Having the right resolution is essential to model explainability because not every application needs the same level of scrutiny.
Transparent AI models offer lenders tools for fair lending upkeep
While it’s always been the case, with the CFPB’s recent guidance and clarification, lenders using advanced algorithms to make credit decisions must provide accurate and specific reason codes to consumers pursuant to the Equal Credit Opportunity Act and Regulation B.
Lenders must be equipped with the right tools to provide precise documentation for AI-automated underwriting models. Documentation like our auto-generated Model Development Model Risk Management (MRM) reports spell out how a model was built, the data on which it was trained, where its data came from, and its validation processes. This level of detail in documentation is key to explainability and also the basis for the compliant use of AI in lending.
All in the name of fair lending…
Explainability and compliance are ultimately the backbone of fair lending. We at Zest AI are committed to equipping lenders with the tools and knowledge they need to operate underwriting models that create consistent, accurate, and most of all fair outcomes for their borrowers. With the right tools, data, and guidance, AI-automated underwriting models can be fully transparent to those who implement them.